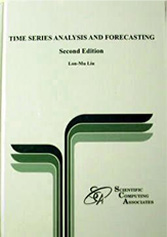
(April 09, Version 2.2)
By Lon-Mu Liu, Ph.D., Univ. of Illinois at Chicago
ISBN: 978-0-9765056-8-6 Format: Hardcover; 578 pp
This book is one of the most important works written on applied time series analysis and forecasting. The author achieves this goal by distilling and integrating important research results on time series analysis and forecasting into cohesive and comprehensible methodologies.
By Lon-Mu Liu, Ph.D., Univ. of Illinois at Chicago
ISBN: 978-0-9765056-8-6 Format: Hardcover; 578 pp
This book is one of the most important works written on applied time series analysis and forecasting. The author achieves this goal by distilling and integrating important research results on time series analysis and forecasting into cohesive and comprehensible methodologies.
Table of Contents
References
Types of a time series
Applications of time series analysis
Approaches for time series analysis and its applications
Model building and forecasting
Principle of parsimony
Automatic model identification approaches
Evaluation of forecast performance
Effects of outliers on forecast performance
References
Stationary time series and their characterization
Autocorrelation function
Partial autocorrelation function
Extended autocorrelation function
Stationary time series models and their characteristics
Autoregressive, moving average, and mixed ARMA models
Nonstationary time series models and their characteristics
Model building
Model identification
Model estimation
Diagnostic checking
Forecasting
Illustrative examples
References
Attributes of seasonal time series
Stationary seasonal models and their characteristics
Pure seasonal autoregressive, moving average, and mixed ARMA models
Nonstationary seasonal models and their characteristics
Multiplicative and nonmultiplicative seasonal models
Seasonal model identification
Estimation of seasonal models
Diagnostic checking of seasonal models
Forecasting with seasonal models
Illustrative examples
References
Two forms of ARIMA models
Automatic identification of ARIMA models for nonseasonal time series
Automatic identification of ARIMA models for seasonal time series
Identification of seasonal ARIMA models using a filtering method
Illustrative examples
References
Relationship of transfer function models to regression models
Multiple-input transfer function models
Transfer function model identification using the LTF method
Transfer function model estimation
Diagnostic checking transfer function models
Forecasting with transfer function models
Illustrative examples
References
Trading day effects
Holiday effects
Modeling trading day effects using ARIMA models
Modeling holiday effects using ARIMA models
Identification of an ARIMA model for a time series with calendar effects
Illustrative examples
References
Characterizations for intervention effects
Modeling strategies for intervention analysis
Forecasting with an intervention model
Outliers in time series and their types (AO, IO, LS, TC)
Methods for outlier detection and adjustment
An iterative procedure for joint estimation of model parameters and outlier effects
Intervention analysis in the presence of outliers
Forecasting in the presence of outliers
Handling outliers at the end of a time series
Handling missing data in a time series
Illustrative examples
References
Naive and averaging methods
Simple (single) exponential smoothing
Double exponential smoothing
Holt's two parameter exponential smoothing
Winters' additive and multiplicative smoothing methods
General exponential smoothing using seasonal indicators
General exponential smoothing using harmonic functions
Relating exponential smoothing to Box-Jenkins ARIMA models
References
Concepts in data mining
Application of data mining concepts on time series analysis and forecasting
The role of expert system time series modeling in data mining
Time series data mining on electricity loads
An example of time series data mining in business operations
Methodology for data mining and knowledge discovery for time series
Using automatic outlier detection methods as a tool for time series data mining
References
Types of transformations for time series
Power transformation and retransformation
Effects of transformation on forecasts
Debiasing forecasts in retransformation
Procedures for searching the optimal power transformation in forecasting applications
Remarks on power transformation
Illustrative examples
References
Symmetric ARCH, GARCH, IGARCH, and GARCH-M models
Asymmetric GJR-GARCH, EGARCH, and Threshold GARCH models
Non-Normal error distributions based on Student-t, Cauchy, and GED
Measuring volatility leverage effects and risk premium
Illustrative examples
References
Periodic grouping of data based on calendar and threshold values
Model estimation using the weighted method
Time-segmented ARIMA models for seasonal time series
Value-segmented threshold autoregressive (TAR) models
Multiplicative TAR models
Multiplicative threshold ARIMA models
Time-segmented transfer function models
Threshold transfer function models
Handling clustered large outliers by discounting segments of time series data
Illustrative examples
References
Threshold autoregressive (TAR) models
Nonlinearity TAR-F Test
Identification of threshold values
Illustrative examples
References Vector ARMA models Relationship between vector ARMA models and other time series models Characteristics of some vector ARMA models Partial autoregression matrices in vector models Extended cross correlation matrices (ECCM) Building vector ARMA models Forecasting using vector ARMA models Multiplicative seasonal vector ARMA models Eigenvalue-eigenvector analyses in multivariate time series Alternative approaches to modeling multivariate time series Illustrative examples
References Simultaneous transfer function models Structural form and reduced form Models Model building strategy for reduced form STF models Model building strategy for structural form STF models Multivariate time series analysis and forecasting with interventions Econometric modeling using STF models Illustrative examples
References
Dynamic relationships between economic time series
Granger causality
Causality testing using vector ARMA models
A decision tree approach for detecting dynamic relationships
Other procedures for testing causality (Sims test and Haugh test)
Illustrative examples
Copyright © All rights reserved. Scientific Computing Associates Corp.
TEL: +708-771-4567 EMAIL: sca@scausa.com
TEL: +708-771-4567 EMAIL: sca@scausa.com